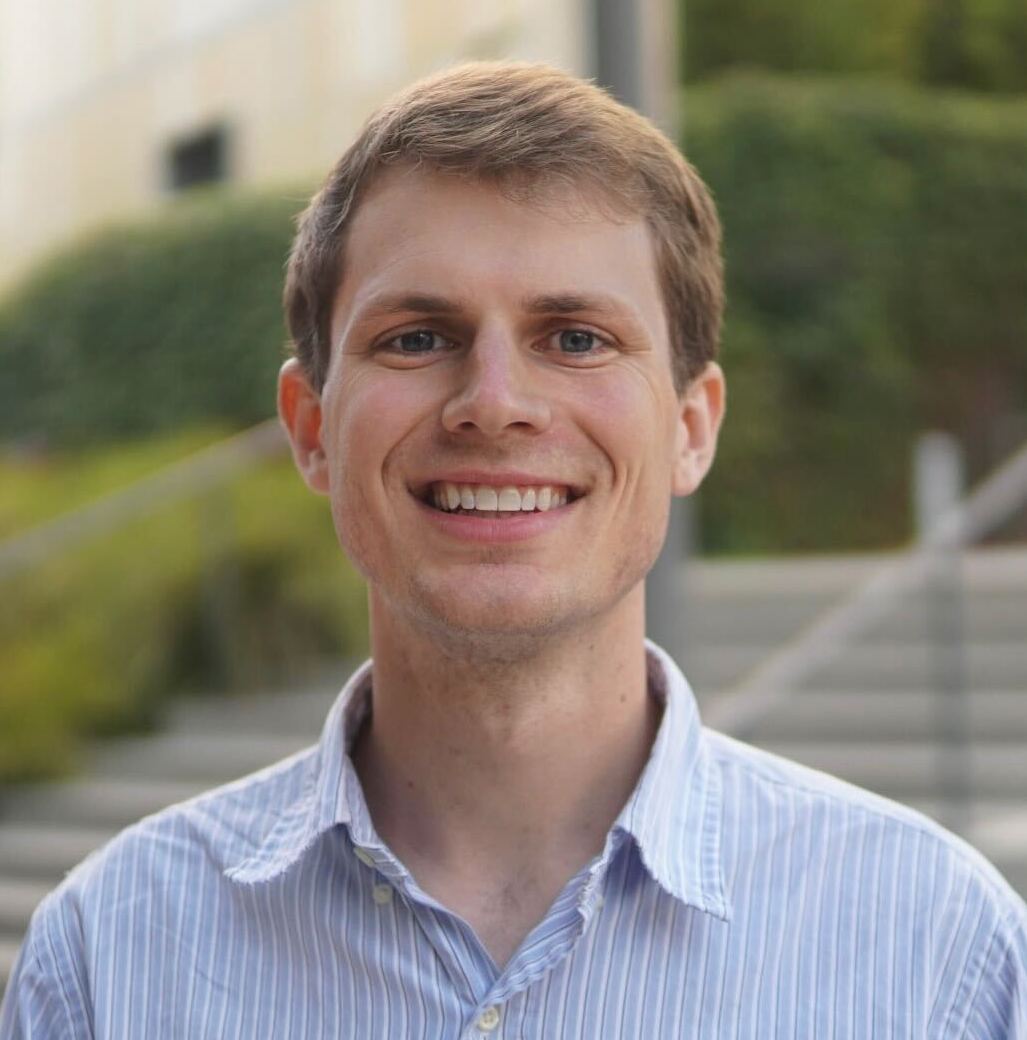
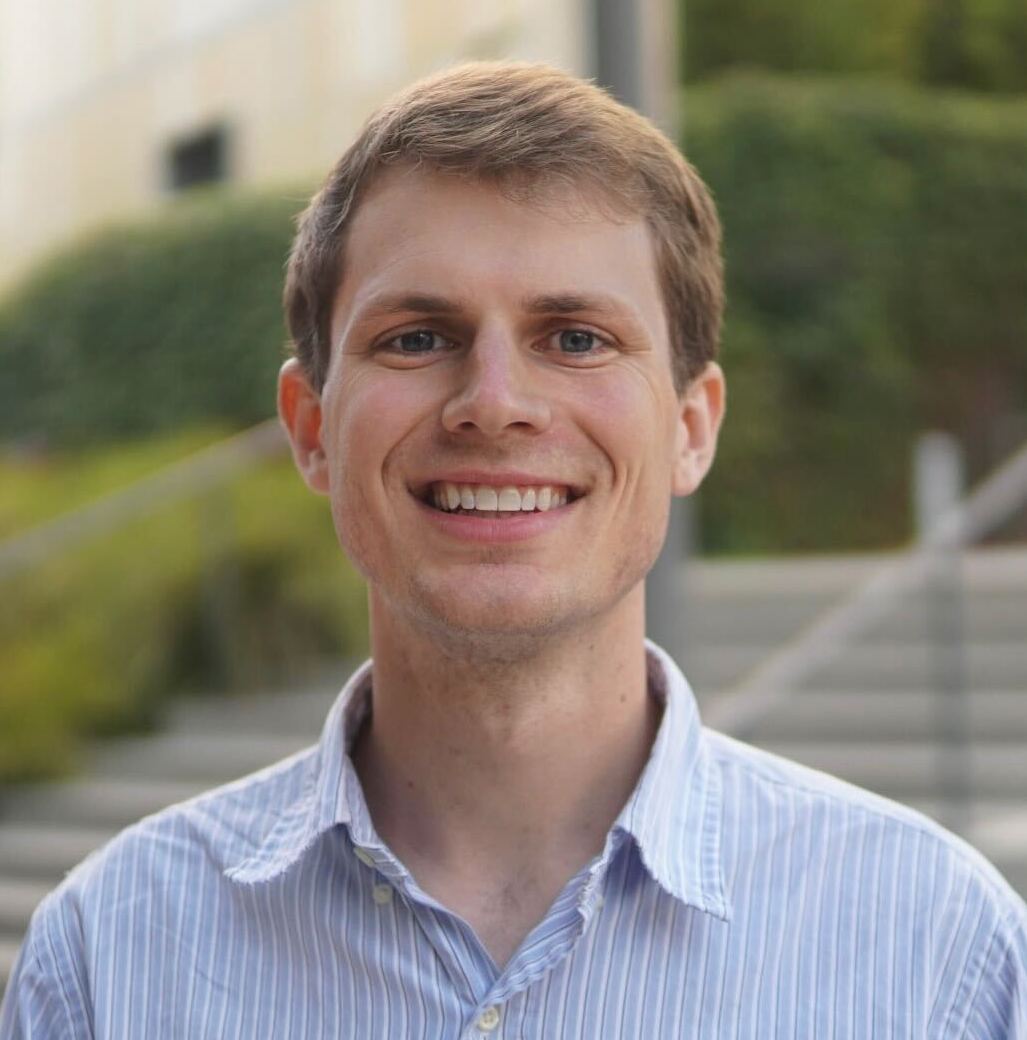
Hello! I am a researcher at OpenAI, where I work to make the next-generation of LLMs more trustworthy, robust, and private. I am currently wrapping up my PhD at UC Berkeley, where I am advised by Dan Klein and Dawn Song.
Hello! I am a researcher at OpenAI, where I work to make the next-generation of LLMs more trustworthy, robust, and private. I am currently wrapping up my PhD at UC Berkeley, where I am advised by Dan Klein and Dawn Song.
Feel free to reach out via email if you are looking to disclose vulnerabilities of OpenAI models. We are also looking for external red teamers!
Current Research Interests
My PhD research focused on enhancing the security/privacy/robustness of ML, improving large language models, and the intersection of these topics. Some of my recent work includes:
- Memorization & Privacy We've shown that LMs and diffusion models can memorize their training data [1,2,3,4], raising questions regarding privacy, copyright, GDPR statutes, and more.
- Prompting & Decoding We've done some of the early work on prompting LMs, including prompt design [4,5], parameter efficiency [6], and understanding failure modes [7].
- Robustness We've studied natural [8] and adversarial distribution shifts [9,10,11], and we have traced model errors back to quality and diversity issues in the training data [12,13,14,15].
- New Threat Models We've explored and refined new types of adversarial vulnerabilities, including stealing models weights [16,17] and poisoning training sets [18,19].
Selected Publications
Here are a few of my representative papers. See my Google Scholar page for a complete list.
-
Scalable Extraction of Data from (Production) Language Models
arXiv 2023
TLDR: We show that adversaries can extract far more memorized text than previously believed, including from production LLMs like ChatGPT.@article{nasr2023scalable, title={Scalable Extraction of Training Data From (Production) Language Models}, author={Nasr, Milad and Carlini, Nicholas and Hayase, Jonathan and Jagielski, Matthew and Cooper, A Feder and Ippolito, Daphne and Choquette-Choo, Christopher A and Wallace, Eric and Tram{\`e}r, Florian and Lee, Katherine}, journal={arXiv preprint arXiv:2311.17035}, year={2023}}
-
The False Promise of Imitating Proprietary LLMs
ICLR 2024
TLDR: We critically analyze the emerging trend of training open-source LMs to imitate predictions from proprietary LLMs (e.g., Alpaca, Koala, Vicuna).@inproceedings{gudibande2023false, title={The False Promise of Imitating Proprietary {LLMs}}, author={Gudibande, Arnav and Wallace, Eric and Snell, Charlie and Geng, Xinyang and Liu, Hao and Abbeel, Pieter and Levine, Sergey and Song, Dawn}, journal={International Conference on Learning Representations}, year={2024}}
-
Extracting Training Data from Diffusion Models
USENIX 2023
TLDR: We show how to extract hundreds of memorized images from popular diffusion models like Imagen and Stable Diffusion.@inproceedings{carlini2023extracting, title={Extracting training data from diffusion models}, author={Carlini, Nicholas and Hayes, Jamie and Nasr, Milad and Jagielski, Matthew and Sehwag, Vikash and Tram{\`e}r, Florian and Balle, Borja and Ippolito, Daphne and Wallace, Eric}, booktitle={USENIX Security Symposium}, year={2023}}
-
Poisoning Language Models During Instruction Tuning
ICML 2023
TLDR: We show that adversaries can poison training sets to manipulate LLM predictions whenever a desired trigger phrase appears, regardless of the task.@inproceedings{Wan2023Poisoning, Author = {Alexander Wan and Eric Wallace and Sheng Shen and Dan Klein}, Booktitle = {International Conference on Machine Learning}, Year = {2023}, Title = {Poisoning Language Models During Instruction Tuning}}
-
Automated Crossword Solving
ACL 2022. First Superhuman Crossword AI
TLDR: We create an AI for solving crossword puzzles that outperforms the world's best human players.@inproceedings{Wallace2022Crosswords, title={Automated Crossword Solving}, author={Wallace, Eric and Tomlin, Nicholas and Xu, Albert and Yang, Kevin and Pathak, Eshaan and Ginsberg, Matthew L. and Klein, Dan}, booktitle={Association for Computational Linguistics}, year={2022}}
-
Calibrate Before Use: Improving Few-shot Performance of Language Models
ICML 2021. Oral Presentation, top 3%
TLDR: We are the first to show that LLM accuracy highly varies across different prompts. We propose a calibration procedure that mitigates the need for prompt engineering.@inproceedings{Zhao2021Calibrate, Title = {Calibrate Before Use: Improving Few-shot Performance of Language Models}, Author = {Tony Z. Zhao and Eric Wallace and Shi Feng and Dan Klein and Sameer Singh}, booktitle={International Conference on Machine Learning}, Year = {2021}}
-
Extracting Training Data From Large Language Models
USENIX Security 2021. PET Award Runner Up
TLDR: We create a method for extracting verbatim training examples from an LLM.@inproceedings{carlini2020extracting, title={Extracting Training Data from Large Language Models}, author={Nicholas Carlini and Florian Tram\`er and Eric Wallace and Matthew Jagielski and Ariel Herbert-Voss and Katherine Lee and Adam Roberts and Tom Brown and Dawn Song and \'Ulfar Erlingsson and Alina Oprea and Colin Raffel}, booktitle={USENIX Security Symposium}, year={2021}}
-
AutoPrompt: Eliciting Knowledge from Language Models with Automatically Generated Prompts
EMNLP 2020
TLDR: We propose a method for automatically designing prompts for LLMs.@inproceedings{Shin2020Autoprompt, Author = {Taylor Shin and Yasaman Razeghi and Robert L. Logan IV and Eric Wallace and Sameer Singh}, BookTitle={Empirical Methods in Natural Language Processing}, Year = {2020}, Title = {{AutoPrompt}: Eliciting Knowledge from Language Models with Automatically Generated Prompts}}
-
Universal Adversarial Triggers for Attacking and Analyzing NLP
EMNLP 2019
TLDR: We create phrases that cause a model to produce a specific prediction when concatenated to any input. Triggers reveal egregious and insightful errors for text classification, reading comprehension, and text generation.
@inproceedings{Wallace2019Triggers, Author = {Eric Wallace and Shi Feng and Nikhil Kandpal and Matt Gardner and Sameer Singh}, Booktitle = {Empirical Methods in Natural Language Processing}, Year = {2019}, Title = {Universal Adversarial Triggers for Attacking and Analyzing {NLP}}}
-
AllenNLP Interpret: A Framework for Explaining Predictions of NLP Models
EMNLP 2019. Best Demo Award
TLDR: We build an open-source toolkit on top of AllenNLP that makes it easy to interpret NLP models.
@inproceedings{Wallace2019AllenNLP, Author = {Eric Wallace and Jens Tuyls and Junlin Wang and Sanjay Subramanian and Matt Gardner and Sameer Singh}, Booktitle = {Empirical Methods in Natural Language Processing}, Year = {2019}, Title = {{AllenNLP Interpret}: A Framework for Explaining Predictions of {NLP} Models}}
Teaching & Mentoring
I enjoy teaching and mentoring students, and I have been involved with multiple courses at Berkeley.
-
CS188: Intro to AI
UC Berkeley, Summer 2023
-
CS288: Natural Language Processing
UC Berkeley, Spring 2023
-
Interpreting Predictions of NLP Models
EMNLP 2020
-
What a Crossword AI Reveals About Humans
-
Privacy & Security for Diffusion and LMs
-
What does GPT-3 “know” about me?
-
Neil deGrasse Tyson Podcast (Crosswords)
-
Does GPT-2 Know Your Phone Number?
-
AI models spit out photos of people and copyrighted images
-
Privacy Considerations in Language Models
-
Neural Crossword Solver Outperforms Humans For First Time
Selected Media Coverage
Here are a few articles that feature my work, including interviews with my colleagues or myself.